Read Spark Parquet Output Using Python Job Using Arrow
We have a lot of different jobs which run on our Spark Clusters and produce the output in different formats as per the job specifications. Generally the data format that we use are json, parquet and parquet with data partitioning.
There is this one case where we wanted the paquet output data generated by one of the Spark jobs to be used in a standalone Python application for some analytical purpose, so we started exploring the options to do the same and came across Apache Arrow which was best fit for our use case.
Installing Apache Arrow:
If you are using conda/miniconda you can use the following command to install Apache Arrow
conda install -c conda-forge pyarrow
If you want to install the same using PIP then you can use
pip install pyarrow
The following is the data samples that we will be using in this process
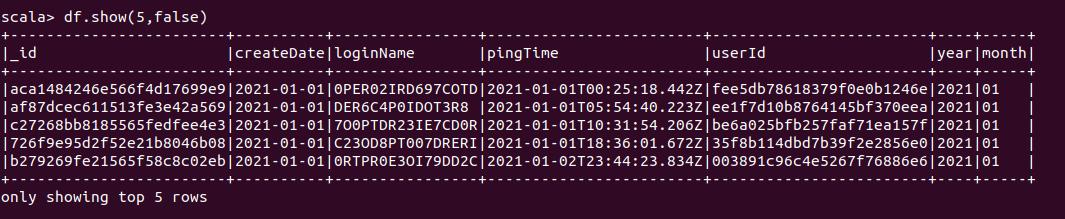
Reading Parquet Data Using Arrow:
The following example will illustrate how to read and use the Spark Parquet output files using standalone Python Job.
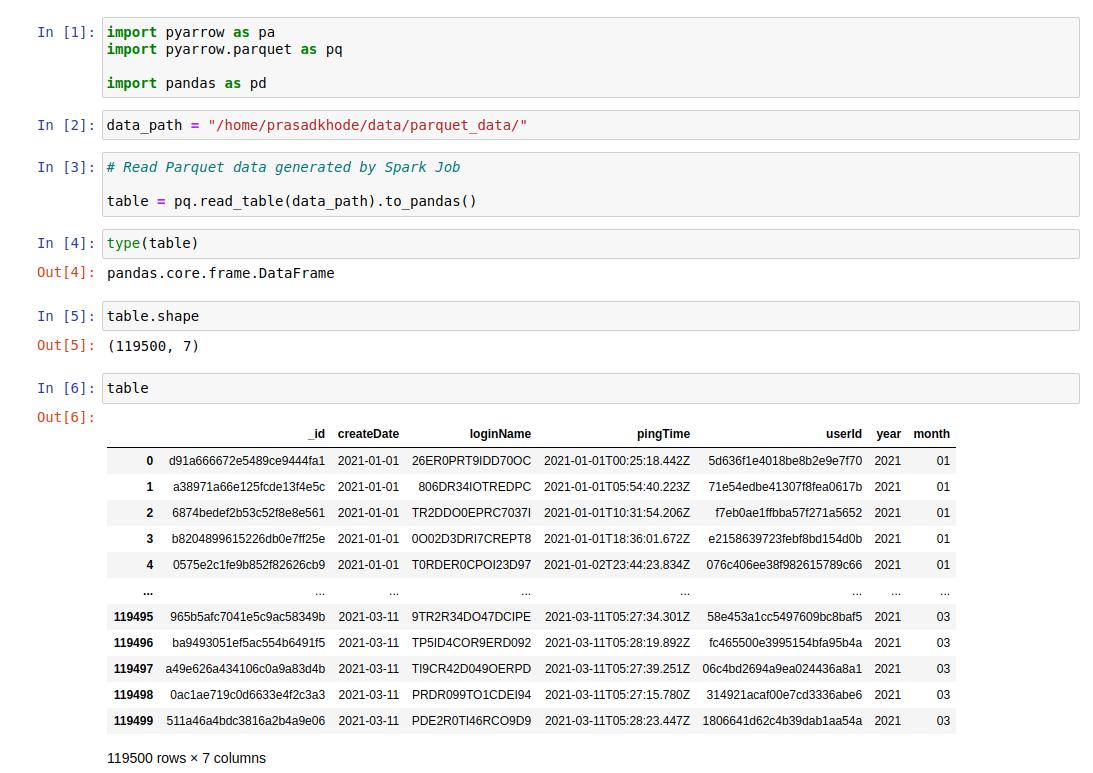
Reading Parquet Partitioned Data Using Arrow:
The following example will illustrate how to read and use Spark Parquet Partitioned data using Python Job.
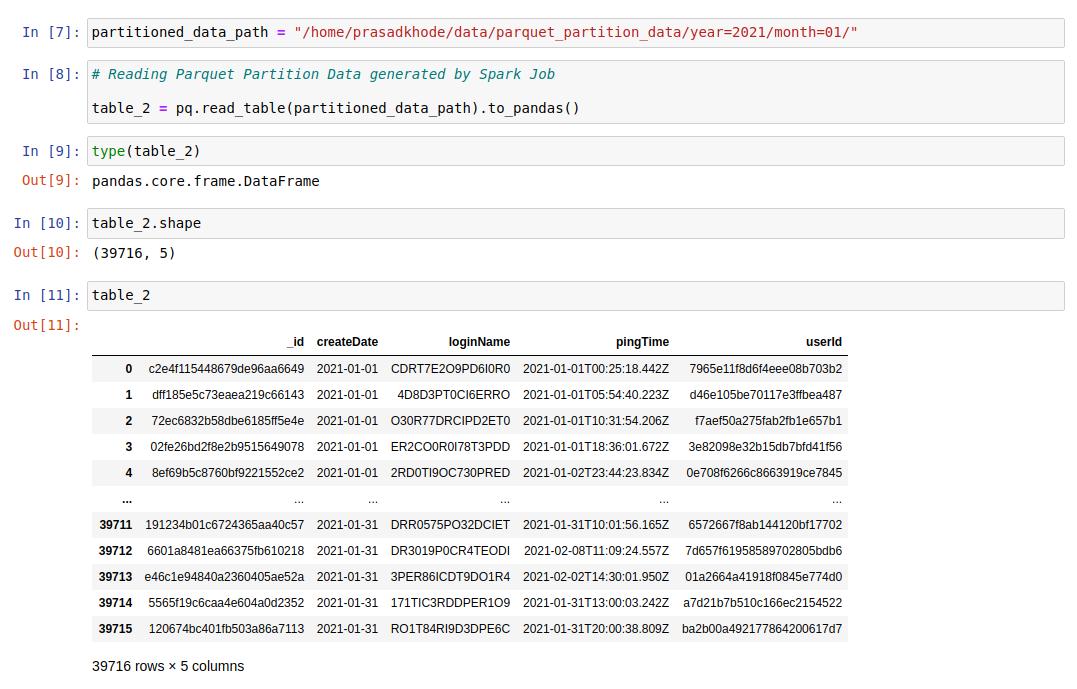
Writing and Reading Data To/From Disk:
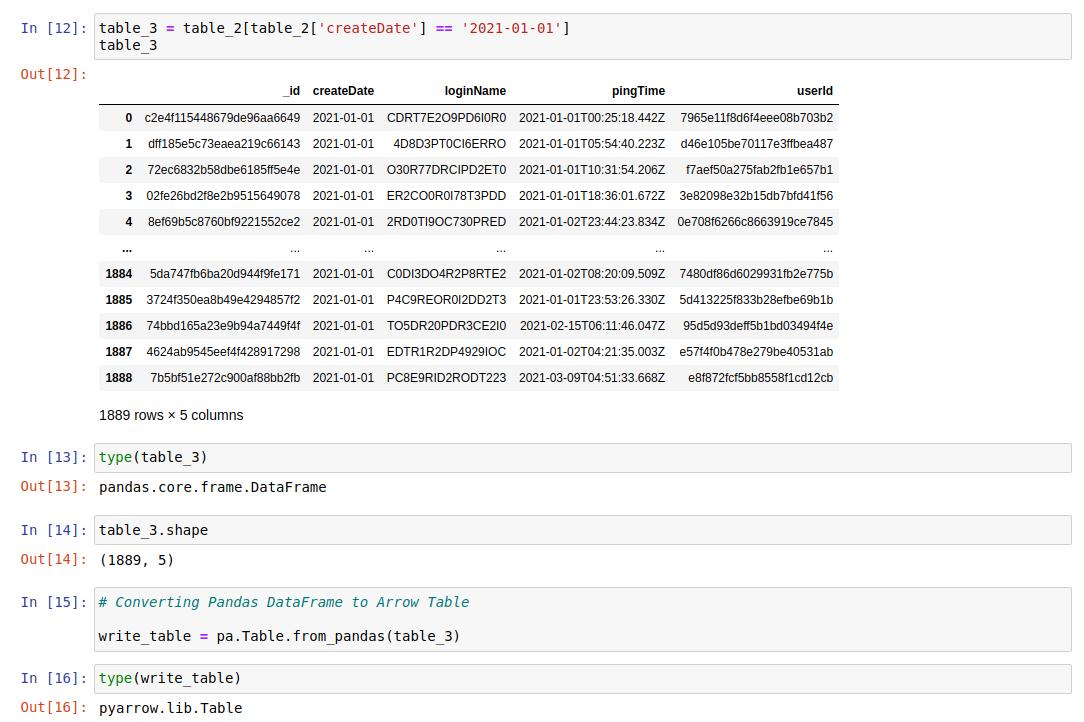
I tried to make this post as simple as it can be, by looking at the code snippet we can understand how to use the Arrow further to read and write the data.
Note:
- The data samples used here are far different from the original use case, this example is used only to illustrate the usage of Apache Arrow
- The data samples used here are masked because of security reasons and does not reflect any of the original data samples.